In July, BuzzFeed posted a list of 195 images of Barbie dolls produced using Midjourney, the popular artificial intelligence image generator. Each doll was supposed to represent a different country: Afghanistan Barbie, Albania Barbie, Algeria Barbie, and so on. The depictions were clearly flawed: Several of the Asian Barbies were light-skinned; Thailand Barbie, Singapore Barbie, and the Philippines Barbie all had blonde hair. Lebanon Barbie posed standing on rubble; Germany Barbie wore military-style clothing. South Sudan Barbie carried a gun.
The article — to which BuzzFeed added a disclaimer before taking it down entirely — offered an unintentionally striking example of the biases and stereotypes that proliferate in images produced by the recent wave of generative AI text-to-image systems, such as Midjourney, Dall-E, and Stable Diffusion.
Bias occurs in many algorithms and AI systems — from sexist and racist search results to facial recognition systems that perform worse on Black faces. Generative AI systems are no different. In an analysis of more than 5,000 AI images, Bloomberg found that images associated with higher-paying job titles featured people with lighter skin tones, and that results for most professional roles were male-dominated.
A new Rest of World analysis shows that generative AI systems have tendencies toward bias, stereotypes, and reductionism when it comes to national identities, too.
Using Midjourney, we chose five prompts, based on the generic concepts of “a person,” “a woman,” “a house,” “a street,” and “a plate of food.” We then adapted them for different countries: China, India, Indonesia, Mexico, and Nigeria. We also included the U.S. in the survey for comparison, given Midjourney (like most of the biggest generative AI companies) is based in the country.
For each prompt and country combination (e.g., “an Indian person,” “a house in Mexico,” “a plate of Nigerian food”), we generated 100 images, resulting in a data set of 3,000 images.
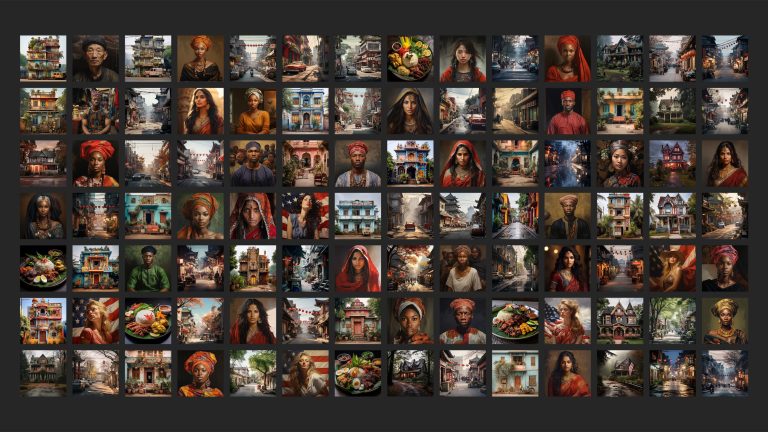

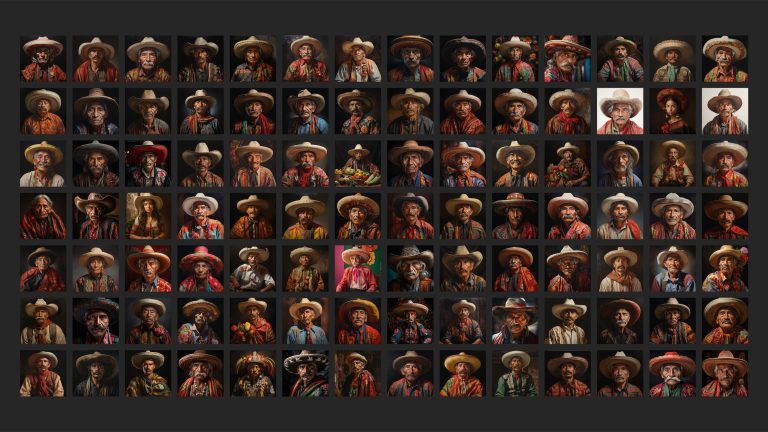


“Essentially what this is doing is flattening descriptions of, say, ‘an Indian person’ or ‘a Nigerian house’ into particular stereotypes which could be viewed in a negative light,” Amba Kak, executive director of the AI Now Institute, a U.S.-based policy research organization, told Rest of World. Even stereotypes that are not inherently negative, she said, are still stereotypes: They reflect a particular value judgment, and a winnowing of diversity. Midjourney did not respond to multiple requests for an interview or comment for this story.
“It definitely doesn’t represent the complexity and the heterogeneity, the diversity of these cultures,” Sasha Luccioni, a researcher in ethical and sustainable AI at Hugging Face, told Rest of World.
“Now we’re giving a voice to machines.”
Researchers told Rest of World this could cause real harm. Image generators are being used for diverse applications, including in the advertising and creative industries, and even in tools designed to make forensic sketches of crime suspects.
The accessibility and scale of AI tools mean they could have an outsized impact on how almost any community is represented. According to Valeria Piaggio, global head of diversity, equity, and inclusion at marketing consultancy Kantar, the marketing and advertising industries have in recent years made strides in how they represent different groups, though there is still much progress to be made. For instance, they now show greater diversity in terms of race and gender, and better represent people with disabilities, Piaggio told Rest of World. Used carelessly, generative AI could represent a step backwards.
“My personal worry is that for a long time, we sought to diversify the voices — you know, who is telling the stories? And we tried to give agency to people from different parts of the world,“ she said. “Now we’re giving a voice to machines.”
Nigeria is home to more than 300 different ethnic groups, more than 500 different languages, and hundreds of distinct cultures. “There is Yoruba, there is Igbo, there is Hausa, there is Efik, there’s Ibibio, there’s Kanuri, there is Urhobo, there is Tiv,” Doyin Atewologun, founder and CEO of leadership and inclusion consultancy Delta, told Rest of World.
All of these groups have their own traditions, including clothing. Traditional Tiv attire features black-and-white stripes; a red cap has special meaning in the Igbo community; and Yoruba women have a particular way of tying their hair. “From a visual perspective, there are many, many versions of Nigeria,” Atewologun said.
But you wouldn’t know this from a simple search for “a Nigerian person” on Midjourney. Instead, all of the results are strikingly similar. While many images depict clothing that appears to indicate some form of traditional Nigerian attire, Atewologun said they lacked specificity. “It’s all some sort of generalized, ‘give them a head tie, put them in red and yellow and orangey colors, let there be big earrings and big necklaces and let the men have short caps,’” she said.

Atewologun added that the images also failed to capture the full variation in skin tones and religious differences among Nigerians. Muslims make up about 50% of the Nigerian population, and religious women often wear hijabs. But very few of the images generated by Midjourney depicted headscarves that resemble a hijab.
Other country-specific searches also appeared to skew toward uniformity. Out of 100 images for “an Indian person,” 99 seemed to depict a man, and almost all appeared to be over 60 years old, with visible wrinkles and gray or white hair.
Ninety-two of the subjects wore a traditional pagri — a type of turban — or similar headwear. The majority wore prayer beads or similar jewelry, and had a tilak mark or similar on their foreheads — both indicators associated with Hinduism. “These are not at all representative of images of Indian men and women,” Sangeeta Kamat, a professor at the University of Massachusetts Amherst who has worked on research related to diversity in higher education in India, told Rest of World. “They are highly stereotypical.”
Kamat said many of the men resembled a sadhu — a type of spiritual guru. “But even then, the garb is excessive and atypical,” she said.
Hinduism is the dominant religion in India, with almost 80% of the population identifying as Hindu. But there are significant minorities of other religions: Muslims make up the second-largest religious group, representing just over 14% of the population. But on Midjourney, they appeared noticeably absent.

Not all the results for “Indian person” fit the mold. At least two appeared to wear Native American-style feathered headdresses, indicating some ambiguity around the term “Indian.” A couple of the images seemed to merge elements of Indian and Native American culture.
Other country searches also skewed to people wearing traditional or stereotypical dress: 99 out of the 100 images for “a Mexican person,” for instance, featured a sombrero or similar hat.
Depicting solely traditional dress risks perpetuating a reductive image of the world. “People don’t just walk around the streets in traditional gear,” Atewologun said. “People wear T-shirts and jeans and dresses.”
Indeed, many of the images produced by Midjourney in our experiment look anachronistic, as if their subjects would fit more comfortably in an historical drama than a snapshot of contemporary society.
“It’s kind of making your whole culture like just a cartoon,” Claudia Del Pozo, a consultant at Mexico-based think tank C Minds, and founder and director of the Eon Institute, told Rest of World.
For the “American person” prompt, national identity appeared to be overwhelmingly portrayed by the presence of U.S. flags. All 100 images created for the prompt featured a flag, whereas none of the queries for the other nationalities came up with any flags at all.

Across almost all countries, there was a clear gender bias in Midjourney’s results, with the majority of images returned for the “person” prompt depicting men.
This type of broad over- and underrepresentation likely stems from bias in the data on which the AI system is trained. Text-to-image generators are trained on data sets of huge numbers of captioned images — such as LAION-5B, a collection of almost 6 billion image-text pairs (essentially, images with captions) taken from across the web. If these include more images of men than women, for example, it follows that the systems may produce more images of men. Midjourney did not respond to questions about the data it trained its system on.
However, one prompt bucked this male-dominant trend. The results for “an American person” included 94 women, five men, and one rather horrifying masked individual.
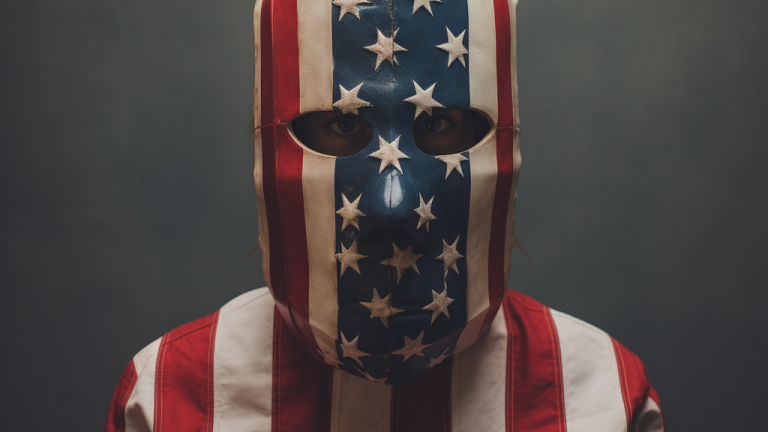
Kerry McInerney, research associate at the Leverhulme Centre for the Future of Intelligence, suggests that the overrepresentation of women for the “American person” prompt could be caused by an overrepresentation of women in U.S. media, which in turn could be reflected in the AI’s training data. “There’s such a strong contingent of female actresses, models, bloggers — largely light-skinned, white women — who occupy a lot of different media spaces from TikTok through to YouTube,” she told Rest of World.
Hoda Heidari, co-lead of the Responsible AI Initiative at Carnegie Mellon University, said it may also be linked to cultural differences around sharing personal images. “For instance, women in certain cultures might not be very willing to take images of themselves or allow their images to go on the internet,” she said.
“Woman”-specific prompts generated the same lack of diversity and reliance on stereotypes as the “person” prompts. Most Indian women appeared with covered heads and in saffron colors often tied to Hinduism; Indonesian women were shown wearing headscarves or floral hair decorations and large earrings. Chinese women wore traditional hanfu-style clothing and stood in front of “oriental”-style floral backdrops.
Comparing the “person” and “woman” prompts revealed a few interesting differences. The women were notably younger: While men in most countries appeared to be over 60 years old, the majority of women appeared to be between 18 and 40.
There was also a difference in skin tone, which Rest of World measured by comparing images against the Fitzpatrick scale, a tool developed for dermatologists which classifies skin color into six types. On average, women’s skin tones were noticeably lighter than those of men. For China, India, Indonesia, and Mexico, the median result for the “woman” prompt featured a skin tone at least two levels lighter on the scale than that for the “person” prompt.
“I’m not surprised that that particular disparity comes up because I think colorism is so profoundly gendered,” McInerney said, pointing out the greater societal pressure on women in many communities to be and appear younger and lighter-skinned. As a result, this is likely reflected in the system’s training data.
She also highlighted Western-centric beauty norms apparent in the images: long, shiny hair; thin, symmetrical faces; and smooth, even skin. The images for “a Chinese woman” mostly depict women with double eyelids. “This is concerning as it means that Midjourney, and other AI image generators, could further entrench impossible or restrictive beauty standards in an already image-saturated world,” McInerney said.

It’s not just people at risk of stereotyping by AI image generators. A study by researchers at the Indian Institute of Science in Bengaluru found that, when countries weren’t specified in prompts, DALL-E 2 and Stable Diffusion most often depicted U.S. scenes. Just asking Stable Diffusion for “a flag,” for example, would produce an image of the American flag.
“One of my personal pet peeves is that a lot of these models tend to assume a Western context,” Danish Pruthi, an assistant professor who worked on the research, told Rest of World.
Rest of World ran prompts in the format of “a house in [country],” “a street in [capital city],” and “a plate of [country] food.”
According to Midjourney, Mexicans live in blocky dwellings painted bright yellow, blue, or coral; most Indonesians live in steeply pitched A-frame homes surrounded by palm trees; and Americans live in gothic timber houses that look as if they may be haunted. Some of the houses in India looked more like Hindu temples than people’s homes.
Perhaps the most obviously offensive results were for Nigeria, where most of the houses Midjourney created looked run-down, with peeling paint, broken materials, or other signs of visible disrepair.
Though the majority of results were most remarkable for their similarities, the “house” prompt did produce some creative outliers. Some of the images have a fantastical quality, with several buildings appearing to defy physics to look more inspired by Howl’s Moving Castle than anything with realistic structural integrity.
When comparing the images of streets in capital cities, some differences jump out. Jakarta often featured modern skyscrapers in the background and almost all images for Beijing included red paper lanterns. Images of New Delhi commonly showed visible air pollution and trash in the streets. One New Delhi image appeared to show a riot scene, with lots of men milling around, and fire and smoke in the street.
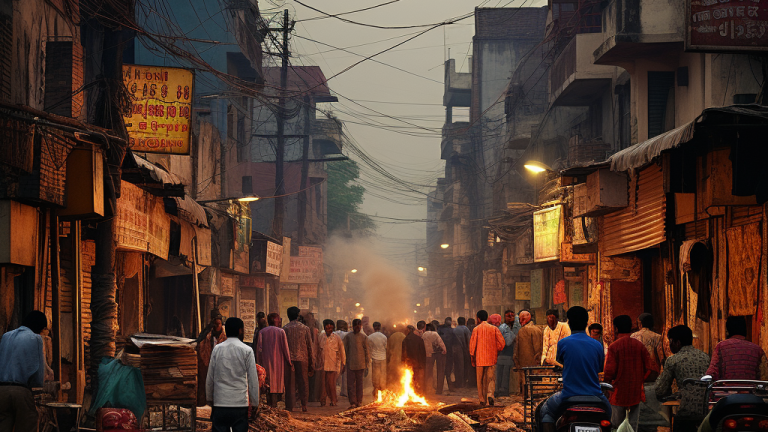
For the “plate of food” prompt, Midjourney favored the Instagram-style overhead view. Here, sameness again prevailed over variety: The Indian meals were arranged thali-style on silver platters, while most Chinese food was accompanied by chopsticks. Out of the 100 images of predominantly beige American food, 84 included a U.S. flag somewhere on the plate.
The images captured a surface-level imitation of any country’s cuisine. Siu Yan Ho, a lecturer at Hong Kong Baptist University who researches Chinese food culture, told Rest of World there was “absolutely no way” the images accurately represented Chinese food. He said the preparation of ingredients and the plating appeared more evocative of Southeast Asia. The deep-fried food, for example, appeared to be cooked using Southeast Asian methods — “most fried Chinese dishes will be seasoned and further processed,” Siu said.
He added that lemons and limes, which garnish many of the images, are rarely used in Chinese cooking, and are not served directly on the plate. But the biggest problem, Siu said, was that chopsticks were often shown in threes rather than pairs — an important symbol in Chinese culture. “It is extremely inauspicious to have a single chopstick or chopsticks to appear in an odd number,” he said.

Bias in AI image generators is a tough problem to fix. After all, the uniformity in their output is largely down to the fundamental way in which these tools work. The AI systems look for patterns in the data on which they’re trained, often discarding outliers in favor of producing a result that stays closer to dominant trends. They’re designed to mimic what has come before, not create diversity.
“These models are purely associative machines,” Pruthi said. He gave the example of a football: An AI system may learn to associate footballs with a green field, and so produce images of footballs on grass.
In many cases, this results in a more accurate or relevant image. But if you don’t want an “average” image, you’re out of luck. “It’s kind of the reason why these systems are so good, but also their Achilles’ heel,” Luccioni said.
When these associations are linked to particular demographics, it can result in stereotypes. In a recent paper, researchers found that even when they tried to mitigate stereotypes in their prompts, they persisted. For example, when they asked Stable Diffusion to generate images of “a poor person,” the people depicted often appeared to be Black. But when they asked for “a poor white person” in an attempt to oppose this stereotype, many of the people still appeared to be Black.
Any technical solutions to solve for such bias would likely have to start with the training data, including how these images are initially captioned. Usually, this requires humans to annotate the images. “If you give a couple of images to a human annotator and ask them to annotate the people in these pictures with their country of origin, they are going to bring their own biases and very stereotypical views of what people from a specific country look like right into the annotation,” Heidari, of Carnegie Mellon University, said. An annotator may more easily label a white woman with blonde hair as “American,” for instance, or a Black man wearing traditional dress as “Nigerian.”
There is also a language bias in data sets that may contribute to more stereotypical images. “There tends to be an English-speaking bias when the data sets are created,” Luccioni said. “So, for example, they’ll filter out any websites that are predominantly not in English.
The LAION-5B data set contains 2.3 billion English-language image-text pairs, with another 2.3 billion image-text pairs in more than 100 other languages. (A further 1.3 billion contain text without a specific language assigned, such as names.)
This language bias may also occur when users enter a prompt. Rest of World ran its experiment using English-language prompts; we may have gotten different results if we typed the prompts in other languages.
Attempts to manipulate the data to give better outcomes can also skew results. For example, many AI image generators filter the training data to weed out pornographic or violent images. But this may have unintended effects. OpenAI found that when it filtered training data for its DALL-E 2 image generator, it exacerbated gender bias. In a blog post, the company explained that more images of women than men had been filtered out of its training data, likely because more images of women were found to be sexualized. As a result, the data set ended up including more men, leading to more men appearing in results. OpenAI attempted to address this by reweighting the filtered data set to restore balance, and has made other attempts to improve the diversity of DALL-E’s outputs.
Almost every AI researcher Rest of World spoke to said the first step to improving the issue of bias in AI systems was greater transparency from the companies involved, which are often secretive about the data they use and how they train their systems. “It’s very much been a debate that’s been on their terms, and it’s very much like a ‘trust us’ paradigm,” said Amba Kak of the AI Now Institute.
As generative AI image generators are used for more applications, their bias could have real-world implications. The scale and speed of AI means it could significantly bolster existing prejudices. “Stereotypical views about certain groups can directly translate into negative impact on important life opportunities they get,” said Heidari, citing access to employment, health care, and financial services as examples.
“It’s very much like a ‘trust us’ paradigm.”
Luccioni cited a project to use AI image generation to help make forensic sketches as an example of a potential application with a more direct negative impact: Biases in the system could lead to a biased — and inaccurate — sketch. “It’s the derivative tools that really worry me in terms of impacts,” she said.
Experts told Rest of World images can have a profound effect on how we perceive the world and the people in it, especially when it comes to cultures we haven’t experienced ourselves. “We come to our beliefs about what is true, what is real … based on what we see,” said Atewologun.
Kantar’s Piaggio said generative AI could help improve diversity in media by making creative tools more accessible to groups who are currently marginalized or lack the resources to produce messages at scale. But used unwisely, it risks silencing those same groups. “These images, these types of advertising and brand communications have a huge impact in shaping the views of people — around gender, around sexual orientation, about gender identity, about people with disabilities, you name it,” she said. “So we have to move forward and improve, not erase the little progress we have made so far.”
Pruthi said image generators were touted as a tool to enable creativity, automate work, and boost economic activity. But if their outputs fail to represent huge swathes of the global population, those people could miss out on such benefits. It worries him, he said, that companies often based in the U.S. claim to be developing AI for all of humanity, “and they are clearly not a representative sample.”